Are you up to building a simple intelligent chatbot? Creating a chatbot that leverages Meta’s Llama 2 model to intelligently query banking data stored in Excel involves several key steps. This guide will walk you through the process, from setting up your environment to deploying your chatbot.
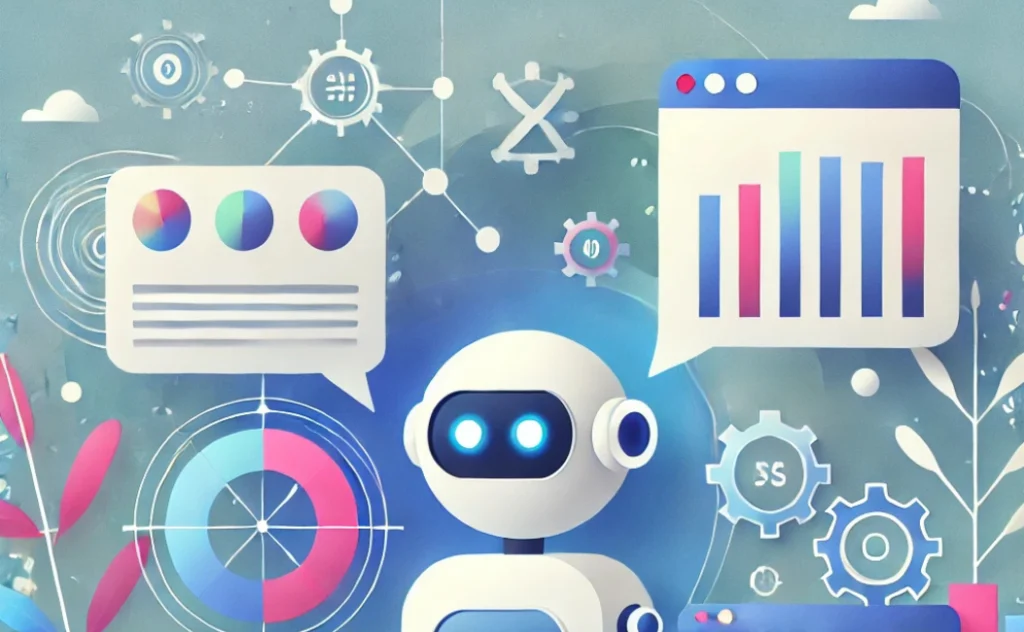
Prerequisites to Build an Intelligent Banking Chatbot Using Llama 2
- Programming Knowledge: Intermediate proficiency in Python.
- Computational Resources: A machine with a GPU is recommended for running large language models efficiently.
- Data Preparation: An Excel file (
.xlsx
) containing your banking data. - Ethical Considerations: Awareness of data privacy laws and ethical AI usage, especially when handling sensitive banking information.
Step 1: Set Up Your Development Environment
Install Python Packages
Begin by installing the necessary Python libraries:
pip install pandas transformers torch sentencepiece accelerate langchain
- pandas: For data manipulation.
- transformers: To access Llama 2 via Hugging Face.
- torch: PyTorch backend for model computations.
- sentencepiece: Tokenization library required by Llama 2.
- accelerate: For optimized model loading.
- langchain: Simplifies interaction with language models.
Step 2: Obtain and Configure Llama 2
Access the Model
Llama 2 is available through the Hugging Face Hub. You need to accept the model’s license on Hugging Face before downloading.
from transformers import AutoTokenizer, AutoModelForCausalLM
model_name = "meta-llama/Llama-2-7b-chat-hf" # You can choose larger models if resources allow
tokenizer = AutoTokenizer.from_pretrained(model_name)
model = AutoModelForCausalLM.from_pretrained(
model_name,
load_in_8bit=True, # Reduces memory usage
device_map='auto'
)
Step 3: Load and Preprocess Your Excel Banking Data
Use pandas
to read and preprocess your data:
import pandas as pd
# Load your banking data
df = pd.read_excel('banking_data.xlsx')
# Preprocess data if necessary (e.g., handle missing values)
df.fillna(0, inplace=True)
Step 4: Define a Function to Interpret User Queries
Create a function that uses Llama 2 to convert natural language queries into actionable insights:
def generate_response(query):
prompt = f"""You are a banking assistant chatbot. Use the provided banking data to answer the following question:
Data:
{df.head().to_string()}
Question:
{query}
Answer the question in detail, using the data where necessary."""
inputs = tokenizer(prompt, return_tensors="pt").to('cuda')
outputs = model.generate(**inputs, max_new_tokens=150)
response = tokenizer.decode(outputs[0], skip_special_tokens=True)
return response.split("Answer:")[-1].strip()
Step 5: Implement Secure Code Execution (Optional but Recommended)
If you plan to execute code generated by the model, sandbox the execution to prevent security risks:
import ast
import sys
def safe_eval(expr, df):
# Define allowed names
allowed_names = {"df": df, "pd": pd}
# Parse the expression
node = ast.parse(expr, mode='eval')
# Compile the AST
code = compile(node, '<string>', 'eval')
# Evaluate the expression
return eval(code, {"__builtins__": {}}, allowed_names)
Step 6: Build the Chatbot Interface
Create a simple command-line interface for your chatbot:
def chatbot():
print("Welcome to the Banking Chatbot. Type 'exit' to quit.")
while True:
user_input = input("You: ")
if user_input.lower() in ('exit', 'quit'):
print("Chatbot: Thank you for using the Banking Chatbot. Goodbye!")
break
response = generate_response(user_input)
print(f"Chatbot: {response}\n")
Run the chatbot:
if __name__ == "__main__":
chatbot()
Step 7: Enhance the Chatbot with LangChain (Optional)
LangChain can help manage prompts and chain together LLM calls:
from langchain import PromptTemplate, LLMChain
from langchain.llms import HuggingFacePipeline
# Wrap the model in a pipeline
from transformers import pipeline
pipe = pipeline(
"text-generation",
model=model,
tokenizer=tokenizer,
device=0
)
llm = HuggingFacePipeline(pipeline=pipe)
# Create a prompt template
template = """You are a banking assistant with access to banking data.
Data:
{data}
Question:
{question}
Provide a detailed answer using the data."""
prompt = PromptTemplate(
input_variables=["data", "question"],
template=template,
)
# Create a chain
chain = LLMChain(llm=llm, prompt=prompt)
# Update generate_response function
def generate_response(query):
data_preview = df.head().to_string()
return chain.run(data=data_preview, question=query)
Step 8: Ensure Compliance and Data Security
- Data Anonymization: Remove or mask sensitive information (e.g., account numbers, personal details).
- Secure Storage: Encrypt the Excel file and limit access permissions.
- Compliance: Ensure adherence to regulations like GDPR or HIPAA.
Step 9: Test the Chatbot
Conduct thorough testing:
- Functional Tests: Verify that the chatbot answers questions accurately.
- Performance Tests: Check response times and optimize if necessary.
- Security Tests: Ensure that the chatbot does not expose sensitive data or execute harmful code.
Step 10: Deploy the Chatbot
Choose a deployment method:
- Local Deployment: For personal use or testing.
- Web Application: Use frameworks like Flask or Django to create a web interface.
- Messaging Platforms: Integrate with Slack, Microsoft Teams, or other platforms using their APIs.
Additional Considerations
- Scalability: For large datasets, consider database solutions instead of loading all data into memory.
- User Authentication: Implement authentication mechanisms to restrict access to authorized users.
- Logging: Keep logs for monitoring and debugging, ensuring they are stored securely.
Example Interaction
Welcome to the Banking Chatbot. Type 'exit' to quit.
You: What was the total revenue last quarter?
Chatbot: The total revenue for the last quarter was $1.5 million.
Conclusion
By integrating Llama 2 with your banking Excel data, you’ve built a powerful chatbot capable of understanding and responding to complex queries. Remember to prioritize data security and compliance throughout your development process.
References